Engineering and Computer Science Researchers at Rensselaer Polytechnic Institute To Partner with GE Global Research, Massachusetts General Hospital, and Los Alamos National Lab
September 24, 2012
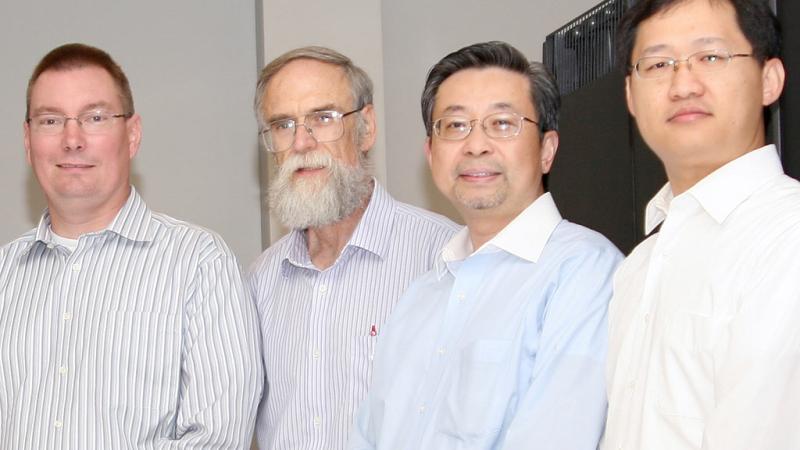
Video gamers are generally the biggest consumers of computer graphics cards, using the devices to boost the speed and resolution of their digital quests to fend off invading extraterrestrials or outwit hostile dragons. But researchers at Rensselaer Polytechnic Institute seek to harness the power of these computer graphics cards to solve one of the world’s most pressing health care technology challenges: radiation exposure from X-ray and CT imaging scans.
Rensselaer nuclear engineering expert X. George Xu is leading an interdisciplinary team of academic, medical, and industrial researchers to develop new techniques for quickly calculating the radiation dose a patient will receive from a CT scan. Funded by a $2.6 million grant from the National Institute of Biomedical Imaging and Bioengineering (NIBIB), the research team aims to use NVIDIA video cards and leading-edge parallel processing techniques to help reduce radiation dose calculations from 10 hours to less than 60 seconds. The team also seeks to test this technology at Massachusetts General Hospital using General Electric’s LightSpeed CT scanners.
“With this new study, we hope to bring massively parallel computing power—currently available only to national laboratories and major research universities such as Rensselaer—to busy and resource-limited hospitals,” said Xu, professor in the Department of Mechanical, Aerospace, and Nuclear Engineering (MANE) and the Department of Biomedical Engineering at Rensselaer, who heads the university’s Nuclear Engineering Program. “There is a high level of interest at the national level to quantify and reduce the amount of ionizing radiation involved in medical imaging. Our parallel computing method has the potential to be used in everyday clinical procedures, which would dramatically decrease the amount of radiation we receive from CT scans.”
Three Rensselaer faculty members are partnering with Xu on this study: Wei Ji, assistant professor in MANE; Christopher Carothers, professor in the Department of Computer Science; and Mark Shephard, the Samuel A. Johnson ’37 and Elisabeth C. Johnson Professor of Engineering and director of the university’s Scientific Computation Research Center. The Rensselaer team will collaborate with radiologist Mannudeep Kalra and medical physicist Bob Liu at Massachusetts General Hospital in Boston, and Paul Fitzgerald from GE Global Research. Additionally, Forrest Brown from Los Alamos National Lab will serve as a consultant to the project.
As part of the study, the research team will perform calculations using the Rensselaer supercomputing center, the Computational Center for Nanotechnology Innovations (CCNI).
Medical imaging scans can provide invaluable information to physicians, and the clinical benefits of a single X-ray or CT scan almost always outweigh the risks of radiation exposure from the procedure. But this radiation exposure risk accumulates over a patient’s lifetime, and is a growing concern given the increased frequency with which CT scans are prescribed and performed.
A 2009 report by the National Council on Radiation Protection and Measurements (NCRP), of which Xu is a member, details how the U.S. population is now exposed to seven times more radiation every year from medical imaging exams than it was in 1980. While CT scans only account for 10 percent of diagnostic radiological exams, the procedure contributes disproportionately—about 67 percent—to the national collective medical radiation exposure.
To help mitigate this risk, several national and international bodies have called for the establishment of a centralized “dose registry” system. Such a system would track over time the number of CT scans a patient undergoes, and the radiation exposure resulting from those procedures. Additional efforts by the radiology community call for new measures to avoid unjustified CT scans and to greatly reduce the radiation exposure for pediatric and pregnant patients. However, current software packages for determining and for tracking CT doses are insufficient for such a critical task, Xu said.
To help solve this problem, Xu has spent nearly a decade developing software that uses highly realistic 3-D virtual reality models—called “computational phantoms”—to calculate the exact amount of radiation a specific organ of the patient will receive from a CT scan. Running on a standard desktop computer, however, the software currently takes about 10 hours to perform the Monte Carlo calculation and produce a result—far too long to be practical in a clinical setting. Monte Carlo simulation, originally developed in the 1940s as an outgrowth of nuclear weapons research, is the only computational method that can provide accurate results for non-uniform 3-D subjects like the human body, Xu said.
In the new $2.6 million study funded by NIBIB, Xu and the research team will design and test new Monte Carlo simulation software to be run on the graphic processing units (GPUs) found in computer graphics cards, instead of running solely on the central processing units (CPUs) of a desktop computer. They have to build the software from scratch, as no existing radiation dose software is compatible with extremely fast processors. GPUs are based on “stream processing” programming, which enables efficient and effective parallel processing. Connecting a small number of these video cards presents an inexpensive option for users in hospitals to tackle this “Big Data” challenge and perform massively parallel computation, Xu said. The research team has published preliminary results showing a single $2,000 GPU card can perform as fast as a 1,000-CPU cluster using an existing Monte Carlo code.
“The high-performance computing community is exploring the role of GPUs in massively parallel supercomputer systems,” Carothers said. “From a computer science perspective, in this project we want to understand the fundamental interplay between the algorithms used in the CT dose software and the underlying hardware architecture of existing and forthcoming GPU processors from NVIDIA and ‘cluster on a chip’ designs from Intel and others. We plan to benchmark these architectures with the CT software application against our IBM Blue Gene/Q supercomputer system at CCNI.”
After developing and validating the software, the research team will integrate it with GE LightSpeed CT scanner models and a library of “phantoms” to estimate patient radiation doses in less than a minute. Finally, to demonstrate and evaluate the technology’s clinical benefits, the research team will perform a series of calculations for typical diagnostic CT scanning protocols of the head, chest, and abdomen at Massachusetts General Hospital. Xu said he is confident this research will one day lead to an ability to perform “patient-specific” dose calculations using innovative image processing tools that do not currently exist.
Nuclear engineers rely on Monte Carlo simulation as an essential research tool. Xu said this new unique hardware/software technology research could open the door to many applications in clinical imaging and radiotherapy practices as well as in the analysis of nuclear reactor systems and the evaluation of safety of nuclear workers.
For more information on Xu’s research at Rensselaer, visit:
- Obese Patients Face Higher Radiation Exposure From CT Scans—But New Technology Can Help
http://news.rpi.edu/update.do?artcenterkey=3018 - Wall Street Journal: Scientists Find Safer Ways To Test Medical Procedures
http://on.wsj.com/HayzNv - Patient Safety: Reducing the Risks of Radiation Exposure From CT Scans and X-Rays
http://news.rpi.edu/update.do?artcenterkey=2876 - Safer, More Accurate Radiation Therapy for Expecting Mothers
http://news.rpi.edu/update.do?artcenterkey=2377 - Rensselaer Radiation Measurement and Dosimetry Group
http://www.rpi.edu/dept/radsafe/public_html/index.htm
For more information on parallel scientific computing research at Rensselaer, visit:
- Scientific Computation Research Center
http://www.scorec.rpi.edu/ - Rensselaer Supercomputing Center: CCNI
https://ccni.rpi.edu - Rensselaer Receives NSF Grant To Install Balanced, Green Supercomputer
http://news.rpi.edu/update.do?artcenterkey=2917 - Providing Competitive Advantage to Industry Through High-Performance Computing
http://news.rpi.edu/update.do?artcenterkey=2929