Existing models show bias, and the complex, high-stakes problem warrants a deeper, multifaceted solution
March 6, 2024
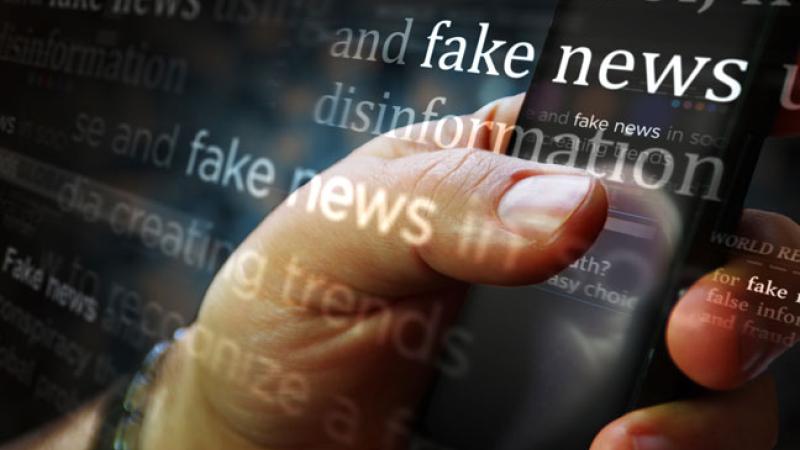
With misinformation and disinformation proliferating online, many may wish for a simple, reliable, automated “fake news” detection system to easily identify falsehoods from truths. Often with the help of machine learning, many scientists have developed such tools, but experts advise caution when deploying them.
In research recently published, Rensselaer Polytechnic Institute’s Dorit Nevo, Ph.D., professor in the Lally School of Management, and colleagues explored the mistakes that these detection tools make. They found that bias and generalizability are challenges because of the models’ training and design, along with the unpredictability of news content. The challenges give rise to ethical concerns. Nevo was joined in research by Benjamin D. Horne, Ph.D., assistant professor in Data Science and Engineering at the School of Information Sciences at the University of Tennessee, and Susan L. Smith, Ph.D., senior lecturer in Cognitive Science at Rensselaer.
“Models are ranked on performance metrics and only research on the best performing model is published,” say the authors. “This format sacrifices empirical rigor and does not take into account the deployment context.” For example, a model may deem one source as reliable, or true, when the source may in fact publish a mix of true and false news, depending on the topic.
On top of that, a set of labels referred to as ground truth is used to train and evaluate the models, and the people generating the labels may be uncertain themselves whether a news item is real or fake.
Together, these elements may perpetuate biases.
“One consumer may view content as biased that another may think is true,” said Nevo. “Similarly, one model may flag content as unreliable, and another will not. A developer may consider one model the best, but another developer may disagree. We think a clear understanding of these issues must be attained before a model may be considered trustworthy.”
The research team analyzed 140,000 news articles from one month in 2021 and examined the issues that arise from automated content moderation. They came to three main conclusions. First, who chooses the ground truth matters. Second, operationalizing tasks for automation may perpetuate bias. Third, ignoring or simplifying the application context reduces research validity.
“It is critical to employ diverse developers when determining ground truth,” said Horne. “Not only should we employ programmers and data analysts in the task, but also experts in other fields as well as members of the general public.”
Smith adds, “Models have far-reaching societal, economic, and ethical implications that cannot be understood by a single field alone.”
Further, the model must be continually reevaluated. Over time, models may fail to perform as predicted and the ground truth may become uncertain. As anomalies increase, experts must explore new approaches for establishing ground truth. Similarly, the methods for establishing ground truth will evolve as science advances, and so must our models.
Finally, we must understand the severe implications that inaccurate fake news detection would have and consider that a single model may never pose a one size fits all solution. Perhaps media literacy combined with a model’s suggestions would offer the most reliability, or a model should be applied to only one news topic as opposed to everything.
“By combining weak, limited solutions, we may be able to create strong, robust, fair, and safe solutions,” the researchers conclude.
“At this point in history, with the rampant spread of misinformation and the polarization of society, stakes could not be higher for developing accurate tools to detect fake news. Clearly, we must proceed with caution, inclusiveness, thoughtfulness, and transparency,” said Chanaka Edirisinghe, Ph.D., acting dean of Rensselaer’s Lally School of Management.