NSF grant to accelerate next-generation environmental barrier coatings
September 29, 2021
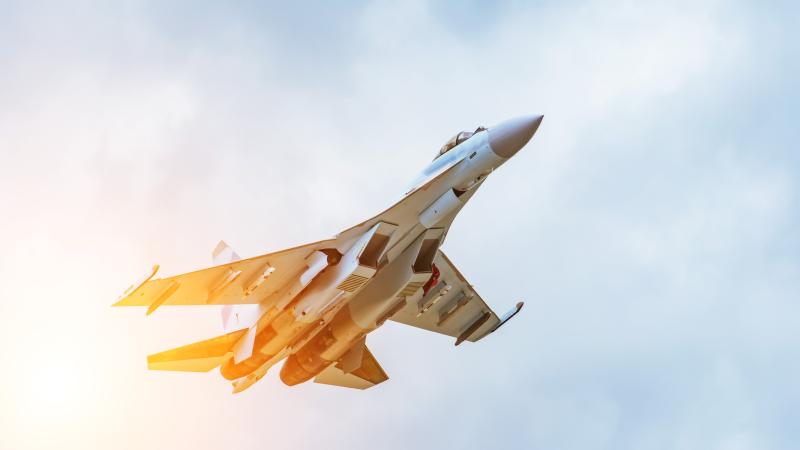
Materials and mechanical scientists are using machine learning to rapidly vet combinations of elements that could be used in next-generation environmental barrier coatings needed to protect vehicles traveling in the extreme conditions of aerospace and space environments. The project, led by researchers at Rensselaer Polytechnic Institute, is supported by the National Science Foundation.
Environmental barrier coatings, or EBCs, are used to seal parts in the engines and structural components of rockets, hypersonic jets, and other space-bound vehicles. The coatings protect parts from harsh operating conditions such as high temperatures, supersonic speeds, intense stress, and severe oxidation and corrosion. Rare earth silicates are the current choice for EBCs used to coat the silicon carbide-based ceramic matrix materials in state-of-the-art jet engines, but these materials are problematic, and subject to performance degradation.
As an alternative, the Rensselaer team proposes EBCs made from multicomponent rare earth phosphates instead of silicates.
“New concepts and innovations are required in order to design next-generation EBCs with transformative performance,” said Jie Lian, a professor in the Department of Mechanical, Aerospace, and Nuclear Engineering and the principal investigator of the grant. “The proposed multicomponent rare-earth phosphates offer unlimited possibilities in designing future EBCs and extending their performance.”
This $1.8 million NSF grant aims to revolutionize material design and support the methodology development by synergizing high-throughput computation – experimentation and machine learning for data-driven materials design and discovery. The researchers will use advanced computer algorithms to create combinations of elements in multiple configurations, determining the most favorable framework for the high-performance EBCs needed for aerospace and space transportation systems of the future.
“The empirical trial-error approach is too expensive and soon becomes impractical for material discovery over a large design space,” said Liping Huang, a co-principal investigator and professor in the Department of Materials Science and Engineering. “We aim at a novel approach that couples physics-based modeling with machine learning to predict the optimal composition and microstructure of the next generation EBCs.”
Lian, an expert in experimentation and material behavior under extreme environments, and Huang, an expert in high-throughput atomic simulation, are joined in this four-year research project by Suvranu De, an expert in finite element analysis and director of the Center for Modeling, Simulation, and Imaging in Medicine (CeMSIM) at Rensselaer, and Lucy Zhang, an expert in machine learning and a professor in the Department of Mechanical, Aerospace, and Nuclear Engineering.
“Machine learning models trained on data generated from high-throughput multiscale simulations can speed up the design and optimization of the structure and performance of multicomponent rare earth phosphates as EBCs,” Zhang said.
The Rensselaer team will collaborate with researchers at the General Electric Global Research, a leading industry of EBCs.