May 19, 2016
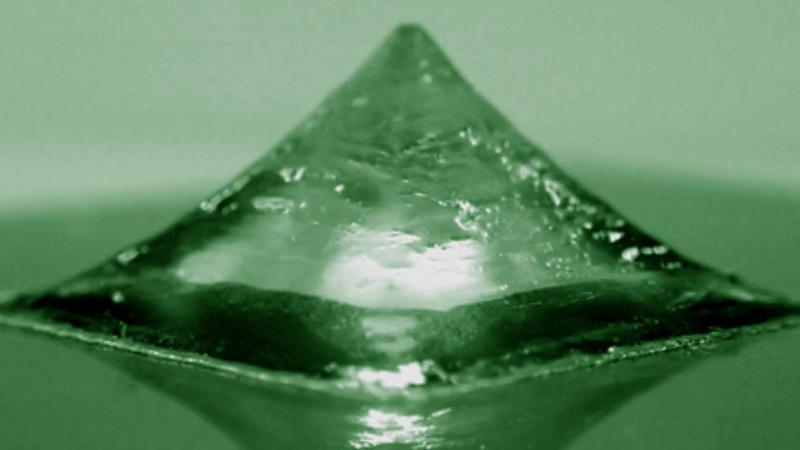

A 3D printed hierarchical fiber-reinforced soft composite part, using technology developed at Rensselaer. Image credit: Johnson Samuel
(This guest posts is part of a series about research related to the Rensselaer IDEA — the Institute for Data Exploration and Applications — a campus-wide institute dedicated to helping researchers navigate the increasingly data-driven landscape of scientific enquiry. In this post, Bryan Chu, who will earn his doctorate in mechanical engineering in May, explains efforts to create modern reference resources for the emerging field of additive manufacturing.)
By now, most people have heard of 3-D printing in some form or another. People and companies making 3-D printed parts ranging from phone cases to cars and houses are frequently in the news. But what most people may not know is that 3-D printing originally referred to a single specific process among a broader class of technologies known as “additive manufacturing.” Every type of additive manufacturing process has the same essential basis; energy is targeted on a small amount of material, which causes localized changes in its properties or phase that enables it to be precisely shaped into the desired geometry. This process continues point-by-point and layer-by-layer to produce whatever geometries are desired. Using this simple operating principle, additive manufacturing techniques can create complex shapes that even the most advanced conventional processes cannot, while using only as much material as is necessary.
Additive manufacturing has been around since the early 1980s, but not long enough to have fully matured as a field. For this reason, trying to transition the manufacturing of a part from conventional machining processes to additive manufacturing can be challenging. One hundred-plus years of manufacturing science and experimentation have been distilled into machining handbooks so that if you walk into a machine shop with a drawing, a machinist can consult one of these books to tell you if and how it can be manufactured.
There’s no equivalent handbook for additive manufacturing. As an emerging field, relevant data does not always exist, or when it does, it is privately held as intellectual property. Data that is available is usually sparse and not easily translated to differing situations.
This is where our project comes in. We want to make a “machining handbook” equivalent for additive manufacturing, and make it freely available, easily usable, and powerful. And in the age of the Internet, we are no longer constrained to just text and numbers on a page.
When someone wants to know how fine they should make their metal powder for a laser-based additive manufacturing process, we want to give them more than a number. We want them to be able to see how the chemistry of the metal, the power of the laser, the motion of the focusing mirrors, and so on, interact to change the process.
We also want to allow them to access the data that backs up those interactions. In this way, when someone develops a new material, a better laser, or even an entirely new additive manufacturing process, they will be able to use that data as a good launching point for building manufacturing processes around the new technology.
Finally, we want to be able to collect and disseminate this data from a centralized, open, and user-friendly data portal and eventually to perform machine learning on our datasets that reveal hidden relations between the materials, equipment, and processes used in additive manufacturing.
Our solution will leverage the power of the semantic web, the “web of data.” We are developing a common framework for recording and compiling additive manufacturing data that can be freely shared and translated across any process or set of equipment. The data will also link intelligently to related pieces of data so that the real-world connections can be encoded. In order to build this framework, we must understand and capture every essential element of the additive manufacturing process and their relations to one another. In data science, the set of all of these elements and relations are formally encoded in what is called an “ontology.”
The idea of an ontology has roots in philosophy, specifically metaphysics, with one example coming from Plato’s Forms. My favorite example of Plato’s Forms involves dogs. What is the ideal “Form” or essence of a dog? A naïve answer might include a furry body connected to four legs and a tail, but where does that leave dogs that are born tailless, or one that may have lost a tail in an accident? Asking and answering these kinds of questions refines and shapes the ontology, but never completes it. Sticking with the dog analogy, eventually we might reach the point of sampling the genome of every type of dog breed to identify all the similarities, but is it necessary to delve that finely?
We face a similar problem in developing the additive manufacturing ontology. We started by considering a few classes of processes, including fused deposition modeling and selective laser sintering. We asked ourselves questions like, “What do the focusing mirrors in selective laser sintering and the mechanical stages in fused deposition modeling have in common?” (The answer is that they both direct energy to the appropriate place.) Many times we would argue down to the definitions of words most people take for granted, like property and input. Eventually we wound up with the definition given in the beginning of this post: energy is targeted at a small amount of material inducing a change so that it can be precisely placed and shaped. Of course, there’s much more than that, including all the equipment, the material properties, and even the machine operator. We are still perfecting the ontology, but the backbone has been solidified in the past year of work.
In order to focus our efforts on the ontology, we have started collecting and generating data to see where there may be gaps. We are collecting the sparse additive manufacturing data that is available from scientific literature. At the same time, the Rensselaer Center for Automation Technologies and Systems is allowing us to use their machines to generate our own data sets. Their systems include a fairly common desktop plastic-based fused deposition device and a large metal powder selective laser sintering machine that they are building in-house. Over the summer, we will also begin designing and programming the back end for the data portal and repository and the front facing website on resources provided by the Tetherless World Constellation (TWC) and based on their successful Deep Carbon Observatory project.

Elements of the Rensselaer CATS laser-based metal additive manufacturing platform. Image credit: Steve Rock
To achieve our goal, we are pooling experts from all over campus. Johnson Samuel, an assistant professor of mechanical, aerospace, and nuclear engineering, and Professor Robert Hull, the director of the Center for Materials, Devices, and Integrated Systems (cMDIS), provide domain-specific expertise in additive manufacturing and materials processing. Professors Peter Fox (chair in the TWC), James Hendler (director of the IDEA, and the TWC Professor of Computer, Web, and Cognitive Sciences), bring experience in semantic web, ontologies, managing big data, and machine learning. Dorit Nevo, an associate professor in the Lally School of Management, is exploring techniques for sourcing and encouraging open dissemination of data from industrial sources.
So far, this work has been supported by Rensselaer through a seed grant from the Office of Research Knowledge and Innovation Program. The current research funding trends point to possible interest from both federal agencies as well as the manufacturing industry. The ultimate goal, though, is to provide an indispensable resource for the exciting nascent field of additive manufacturing and to encourage a culture of openness and data sharing that will be integral to the fourth industrial revolution.