December 3, 2014
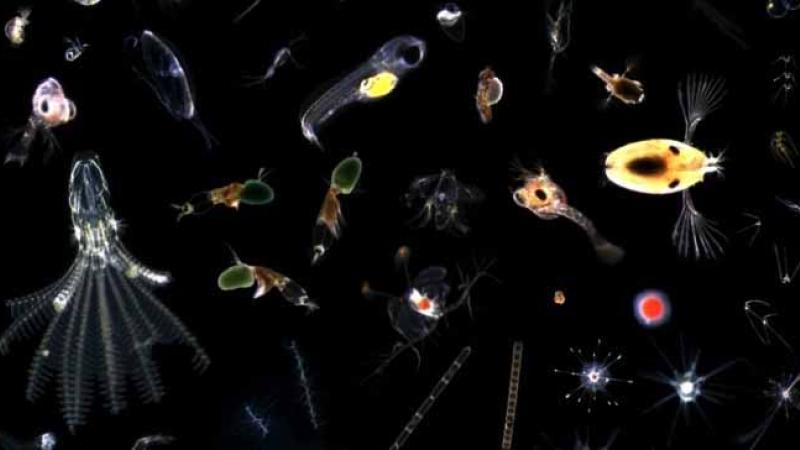
Among my favorite research projects at Rensselaer are two – the Jefferson Project at Lake George, and the Image Based Ecological Information System (IBEIS) – that use data and computation to understand and protect our environment. So my interest was piqued when I heard researchers involved with the two projects were planning a collaboration. A collaboration that, for the purposes of this blog post, I'm calling "Plankton Cam."
The idea behind Plankton Cam is to: tow a specialized camera through the waters of Lake George, capturing more than 100,000 images per day; use advanced pattern recognition software to sort resulting images of phytoplankton by species; and then develop tools to visualize the distribution patterns of the animals at the base of the Lake George food web. The camera, pictured to the left, was developed at the Woods Hole Oceanographic Institute.
When fully realized, Plankton Cam (the proposal's official name is "Building a Three-Dimensional Model of the Plankton Distribution in Lake George") will allow researchers to identify microscopic species at a rate that is, according to the proposal, "orders of magnitude faster than the traditional approach" – in which researchers gather water samples and manually identify species through a microscope.
Plankton Cam is a nearly ideal alignment between IBEIS and the Jefferson Project. IBEIS, a project lead by Chuck Stewart, Rensselaer professor and head of the Department of Computer Science, is developing advanced computational tools that can identify individual animals in tourist photos shot in wildlife sanctuaries. The system is able to automatically process the thousands of images taken by tourists, identifying individuals from several species – such as Grevy's and plains zebras, seals, rhinos, and even lionfish. This information, previously available only by laboriously radio-tagging individual animals, can answer questions about who is where and when, providing invaluable data for research on population, behavior, and stressors on animals.
The Jefferson Project – a collaboration between IBM, RPI, and The FUND for Lake George – combines data analytics and computation with experimentation to understand how human activity impacts Lake George. The project, directed by Rensselaer Professor Rick Relyea, uses data –such as information collected from a network of sensors now being deployed in the lake and its tributaries – to inform a series of computational models that accurately depict processes like the circulation of water, nutrients, and pollutants in the lake. Combined with experimentation, these computer models will allow researchers to create alternate realities – visions of a future lake under various scenarios like new invasive species, increased development or decreased use of road salt.
With Plankton Cam, researchers will adapt the IBIES approach to species identification to provide the Jefferson Project with otherwise unavailable information at the interface between the physical and biological processes within the lake. Here's how the project partners, which include professors of biology, computer science, and art, describe the project impact:
In the Jefferson Project, we have sophisticated sensor systems to detect physical and chemical changes over space and time, but there is no available automated technology to identify and track changes in the plankton. Being able to identify species of zooplankton would also allow biologists to identify larval stages of invasive species– such as zebra mussels and Asian clams– to identify invasions into a lake and to map the invaders' abundance throughout a lake. Having real-time measures of phytoplankton would also allow biologists to quantify patterns of plankton abundance that precede harmful algal blooms, which reduce aesthetics for tourism and can produce harmful toxins.
Recently, I tagged along on a trial run of the concept. Researchers from Woods Hole in Massachusetts brought their camera – the "Continuous Plankton Imaging and Classification Sensor" or "CPICS" – to Rensselaer's Darrin Fresh Water Institute on Lake George to capture images of plankton in the lake. CPICS was developed to look at marine snow, and the microscopic imaging system can capture images ranging in size from several centimeters to 100 microns, and take six to 10 images per second.
In and of itself, CPICS is a wonder. The camera uses a telecentric lens, which, unlike a conventional lens, is able to compensate for the difference in perspective that typically renders objects nearer to the lens as larger than objects which are far away.Objects within range of the lens are backlit in an exposure so brief that there is no contribution from ambient light. The resulting images capture microscopic organisms in their natural environment in astonishing clarity and beauty. You can see a sample of the images at the top of this post.
On a bitter cold day, a research vessel towed the camera, mounted on a submersible unit that also included an altimeter, imaging sonar, a flourometer, and CDT (conductivity, temperature, and depth) reader. By accelerating and decelerating the tow boat, they were able to alter the depth of the submersible, moving it up and down through the water column as it advanced. Below decks, researchers monitored incoming images on a laptop computer.
Woods Hole researchers developed the camera for their Ocean Cubes un-manned underwater observatory program. CPICS does use several identification algorithms but, according to the proposal, it has not yet reached full autonomy. Researchers on IBEIS and CPICS, working together, would adapt recent advances in neural network algorithms to Plankton Cam, as they design, implement, train, and test novel identification algorithms specialized to freshwater species of plankton. Adapting IBEIS for plankton "would represent a major step forward in providing aquatic biologists around the world the ability to test long-standing hypotheses and discover new, emergent hypotheses," write the researchers. For example, they write:
Such a capability would allow, for the first time, the ability to document the distribution of millions of zooplankton across a lake, at all depths, and at multiple time points. This four-dimensional data will give us unprecedented insight into the distribution of the plankton, which serve as the base of aquatic food webs and also include invasive species and "indicator" species associated with positive and negative effects on water quality.
Project collaborators were recently awarded a seed grant through the Rensselaer Office of Research "Knowledge and Innovation" program. Over the course of the next 12 months, they will be working together to develop the capabilities of the sensor, as well as interactive 3-D images of the plankton and data visualizations of the planktonic distributions.