December 2, 2019
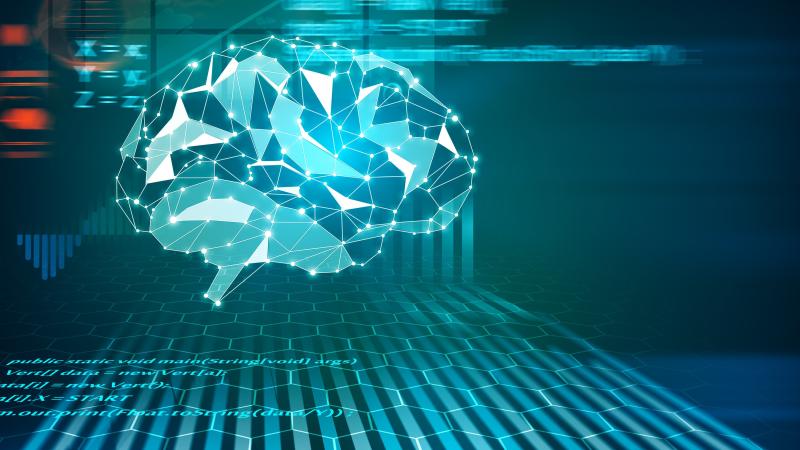
The machine learning techniques that are increasingly being used to interpret data have the potential to advance our understanding and management of human health. Accurate analysis of vast amounts of health-related information could help us learn more about the mechanisms behind certain diseases, identify new ways to treat those diseases, and, in turn, make more informed decisions.
In a position paper recently published in Digital Medicine, a Nature Journal, an interdisciplinary team of experts from across the country lay out a possible path forward for improving these data analytic techniques. The team includes Suvranu De, the J. Erik Jonsson ’22 Distinguished Professor of Engineering and director of the Center for Modeling, Simulation and Imaging in Medicine at Rensselaer Polytechnic Institute.
The authors propose the integration of machine learning, which relies on trained computer algorithms, and multiscale modeling, mathematical methods of solving problems at multiple scales of time and space.
Machine learning integrates different types of data well, while also revealing significant correlations within the data. However, it falls short of recognizing fundamental laws of physics and, in turn, can be unreliable.
Multiscale modeling is effective in integrating multiscale, multiphysics data while uncovering mechanisms that can explain how a particular system functions. It is not effective in combining large datasets from multiple sources.
When combined, the researchers argue, the two approaches can complement one another and more effectively advance research in the fields of biological, biomedical, and behavioral sciences.
In this paper, the researchers also identify challenges that must be addressed in order for the fields to converge, including how to build models when there is insufficient data, how to analyze large amounts of data quickly, and an overall understanding of the limits of machine learning and multiscale modeling.