New technique has the potential to improve the quality and speed of imaging
March 6, 2019
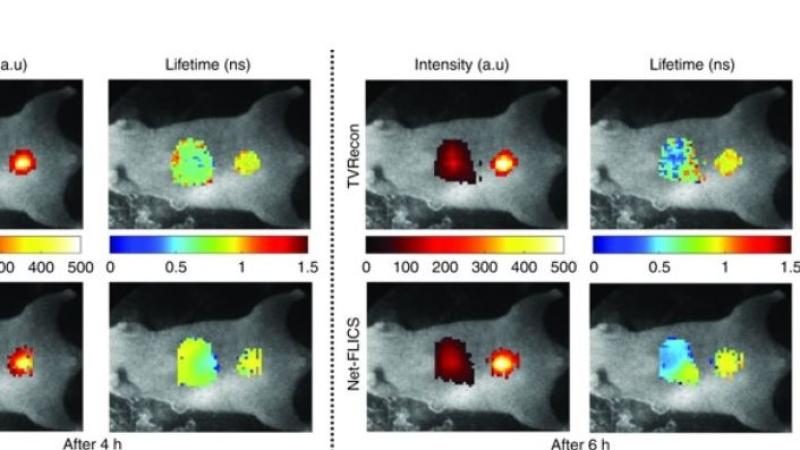
TROY, N.Y. — Generating comprehensive molecular images of organs and tumors in living organisms can be performed at ultra-fast speed using a new deep learning approach to image reconstruction developed by researchers at Rensselaer Polytechnic Institute.
The research team’s new technique has the potential to vastly improve the quality and speed of imaging in live subjects and was the focus of an article recently published in Light: Science and Applications, a Nature journal.
Compressed sensing-based imaging is a signal processing technique that can be used to create images based on a limited set of point measurements. Recently, a Rensselaer research team proposed a novel instrumental approach to leverage this methodology to acquire comprehensive molecular data sets, as reported in Nature Photonics. While that approach produced more complete images, processing the data and forming an image could take hours.
This latest methodology developed at Rensselaer builds on the previous advancement and has the potential to produce real-time images, while also improving the quality and usefulness of the images produced. This could facilitate the development of personalized drugs, improve clinical diagnostics, or identify tissue to be excised.
In addition to providing an overall snapshot of the subject being examined, including the organs or tumors that researchers have visually targeted with the help of florescence, this imaging process can reveal information about the successful intracellular delivery of drugs by measuring the decay rate of the fluorescence.
To enable almost real-time visualization of molecular events, the research team has leveraged the latest developments in artificial intelligence. The vastly improved image reconstruction is accomplished using a deep learning approach. Deep learning is a complex set of algorithms designed to teach a computer to recognize and classify data. Specifically, this team developed a convolutional neural network architecture that the Rensselaer researchers call Net-FLICS, which stands for fluorescence lifetime imaging with compressed sensing.
“This technique is very promising in getting a more accurate diagnosis and treatment,” said Pingkun Yan, co-director of the Biomedical Imaging Center at Rensselaer. “This technology can help a doctor better visualize where a tumor is and its exact size. They can then precisely cut off the tumor instead of cutting a larger part and spare the healthy, normal tissue.”
Yan developed this approach with corresponding author Xavier Intes, the other co-director of the Biomedical Imaging Center at Rensselaer, which is part of the Rensselaer Center for Biotechnology and Interdisciplinary Studies. Doctoral students Marien Ochoa and Ruoyang Yao supported the research.
“At the end, the goal is to translate these to a clinical setting. Usually when you have clinical systems you want to be as fast as possible,” said Ochoa, as she reflected on the speed with which this new technique allows researchers to capture these images.
Further development is required before this groundbreaking new technology can be used in a clinical setting. However, its progress has been accelerated by incorporating simulated data based on modeling, a particular specialty for Intes and his lab.
“For deep learning usually you need a very large amount of data for training, but for this system we don’t have that luxury yet because it’s a very new system,” said Yan.
He said that the team’s research also shows that modeling can innovatively be used in imaging, accurately extending the model to the real experimental data.